Insight
Vision AI Platform Selection Guide: On-Premise vs. Cloud
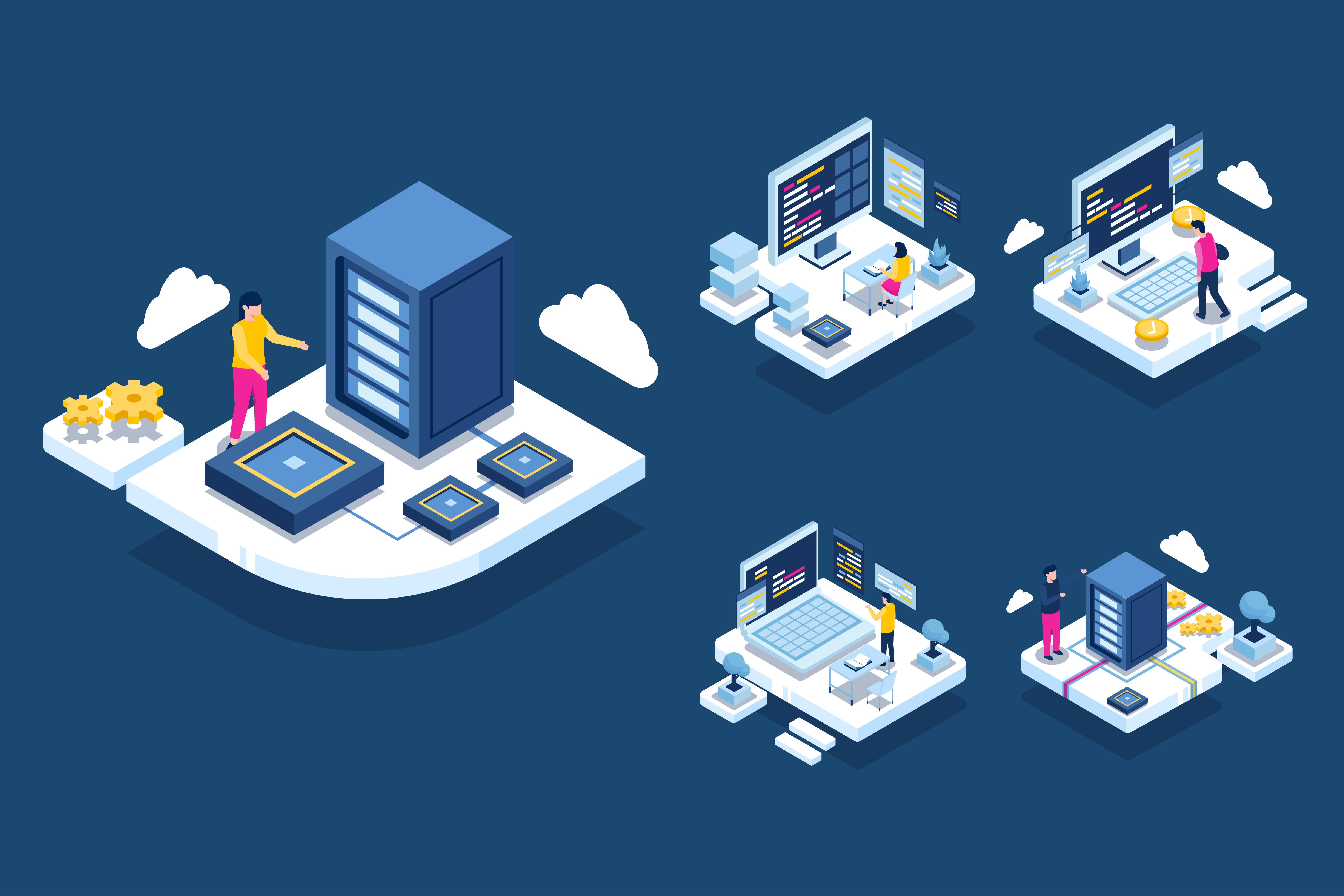
Vision AI Platform Selection Guide: On-Premise vs. Cloud
According to Accenture’s Technology Vision 2025 report, 2025 will be the year when LLMs (Large Language Models) and VLMs (Vision-Language Models) fundamentally reshape robotics. Machine intelligence is expanding into the physical world, and robots are beginning to exhibit reasoning and autonomy. The integration of AI and robotics is expected to revolutionize industries, compelling most enterprises to rethink their physical operations and workflows. The key theme at CES 2025 was also "AI Robots," with NVIDIA CEO Jensen Huang making headlines by stating in his keynote, "The next frontier of AI is 'Physical AI'."
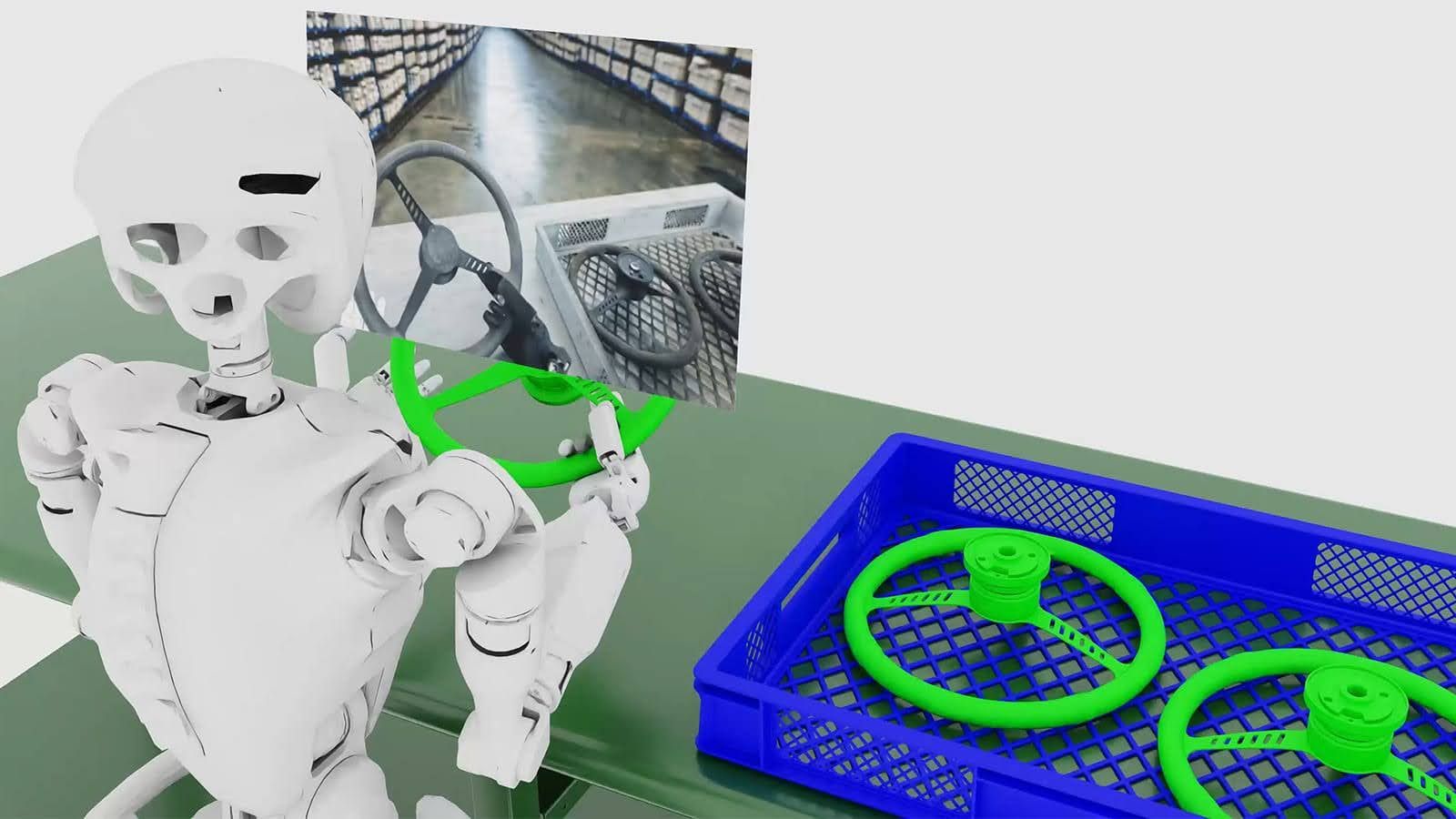
Source : NVIDIA Cosmos
As AI adoption accelerates across industrial settings, or the “physical world,” more companies in heavy industries are inquiring about AI implementation. Yet, a common dilemma is choosing between an on-premise AI platform and a cloud-based AI platform. Given that computer vision AI requires handling large-scale, high-resolution image and video data with real-time processing capabilities, the choice is particularly critical. Drawing on Superb AI’s expertise in vision AI, this guide compares on-premise vs. cloud-based solutions and provides key considerations for selection.
Key Considerations for Vision AI Adoption
Vision AI primarily deals with image and video data, requiring careful evaluation of the following factors:
- Large-scale data processing: High-resolution images and videos generate massive amounts of data, requiring robust processing capabilities, especially in industrial settings or mobile equipment.
- High computing power requirements: Deep learning models demand high-performance computing resources (e.g., GPUs) for training and inference.
- Real-time processing needs: For applications requiring immediate video data analysis (e.g., security monitoring, or production line inspection), ultra-low-latency processing is essential.
- Data security and privacy: Industries handling sensitive data must mitigate risks of data breaches and enforce strict access control.
- Continuous model training and deployment: AI models in dynamic industrial environments must be continuously updated and deployed to adapt to evolving requirements in real-time.
Pros and Cons of “Vision AI + On-Premise”
Pros
- Enhanced data security and privacy: Vision AI often handles sensitive data, and an on-premise environment ensures that data remains within internal servers, minimizing the risk of breaches. This is particularly beneficial for industries like manufacturing, defense, and healthcare, where data security is a top priority.
- Low-latency real-time processing: No network dependency ensures stable performance for real-time analysis of high-resolution video data.
- Customizable infrastructure: Hardware and software configurations can be fully optimized for specific operational requirements.
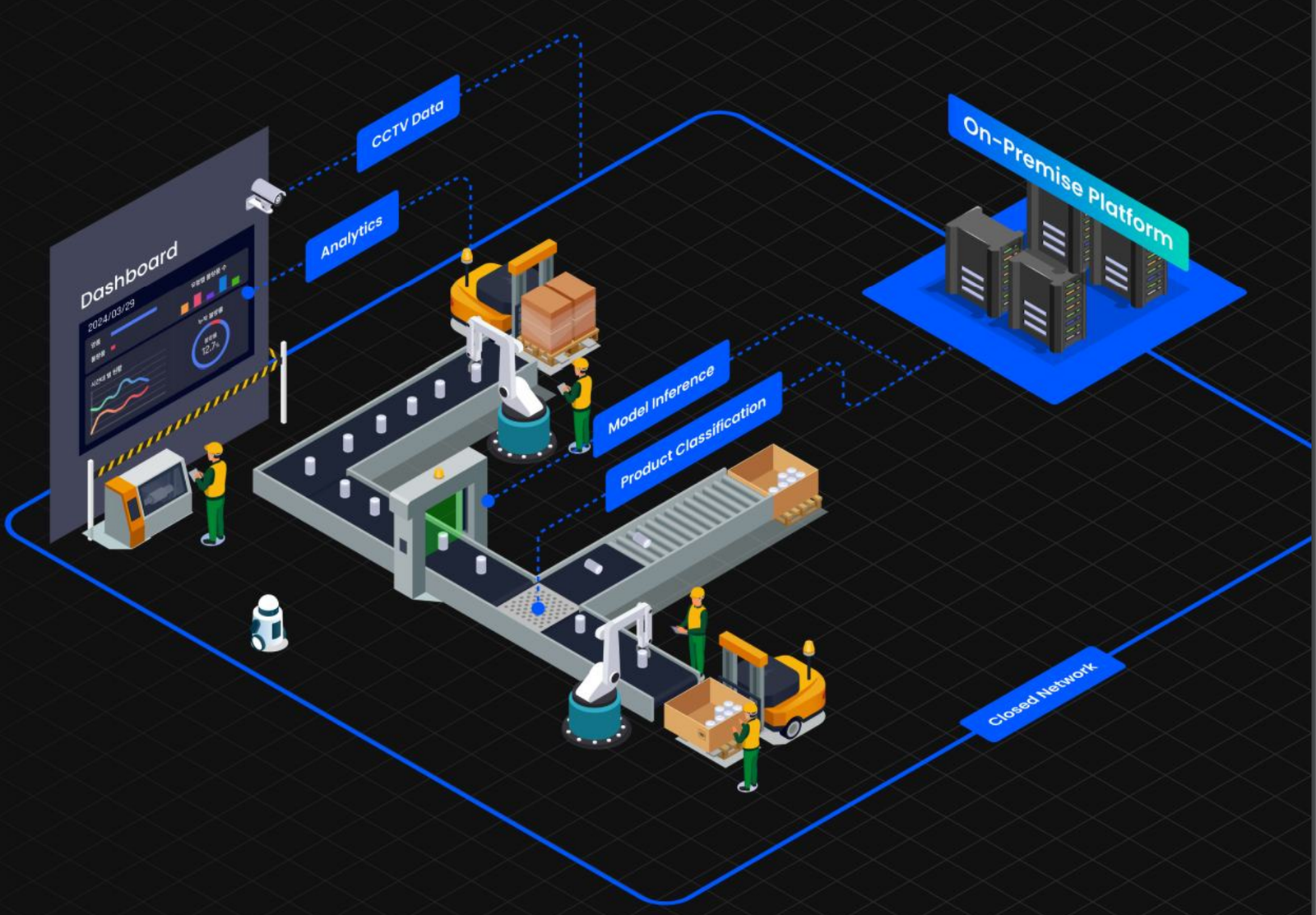
Superb AI On-Premise
Cons
- High initial investment: Setting up and maintaining GPU servers and other high-performance hardware requires significant upfront costs.
- Operational and maintenance burden: If an in-house IT team manages system operations and maintenance, scaling and updating the model may require continuous investment of the team’s time and resources.
- Limited scalability: If data processing demands suddenly increase, expanding resources can be challenging and costly for the on-premise solution.
Pros and Cons of “Vision AI + Cloud”
Pros
- Scalability and flexibility: Cloud-based AI platforms allow businesses to dynamically adapt to different needs and circumstances, especially if they can be integrated with the company’s existing systems.
- Quick deployment and access to the latest technologies: Unlike on-premise solutions that require hardware setup, cloud platforms enable rapid AI adoption and provide continuous access to the latest AI models for continued model advancement.
- No maintenance burden: Cloud providers manage infrastructure and updates, reducing the need for in-house maintenance efforts.
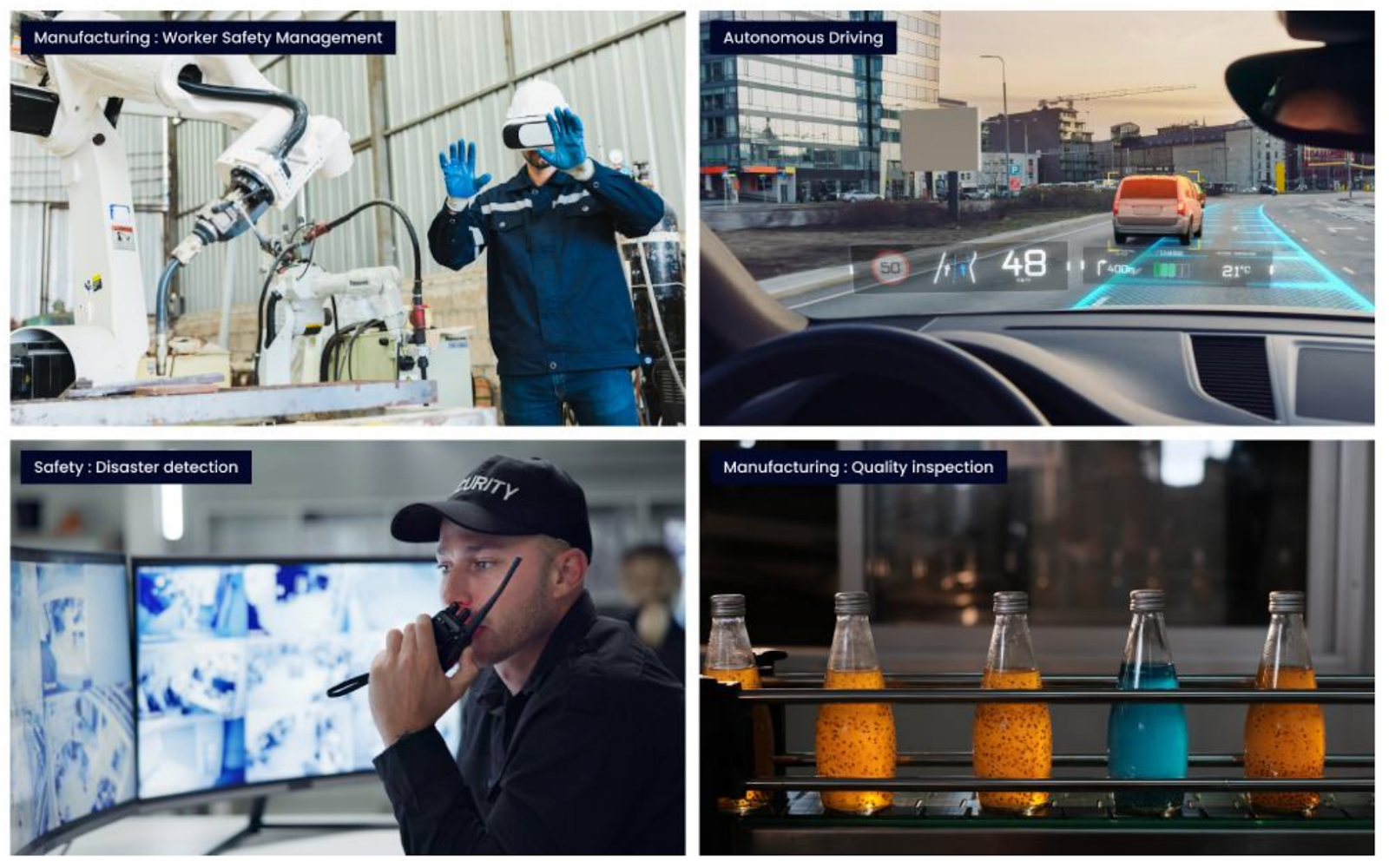
Cons
- Data security concerns: High-resolution video data may contain large amounts of sensitive information, making storage on external servers not an option (e.g., in cases involving personal information or trade secrets).
- Network dependency: If real-time processing of large-scale data is required, network latency may impact AI performance.
- Potential cost increases: As data processing volume increases, costs can rise sharply.
Vision AI Platform Selection Criteria: What to Consider?
Data Security & Privacy
- If handling sensitive video data (e.g., medical imaging, security footage, or classified industrial data), consider adopting an on-premise platform.
- If the data is non-sensitive or security requirements are less stringent, cloud-based options may also be a viable choice.
Processing Speed & Network Dependency
- If the site has no network connection or requires ultra-low-latency real-time processing, on-premise is a better fit.
- If network latency is relatively low or acceptable, a cloud-based solution can be a good option.
Budget & Cost Structure
- If the company can bear the high initial cost, an on-premise solution may be more cost-effective in the long run.
- If the upfront investment is a burden or if a variable cost structure is preferable, a cloud-based solution is a better choice.
Technical Capabilities & Operational Resources
- If the company has sufficient internal IT resources, an on-premise solution can be managed effectively.
- If reducing operational burden is a priority, a cloud-based solution is preferable.
Comparison between On-Premise vs Cloud Platform
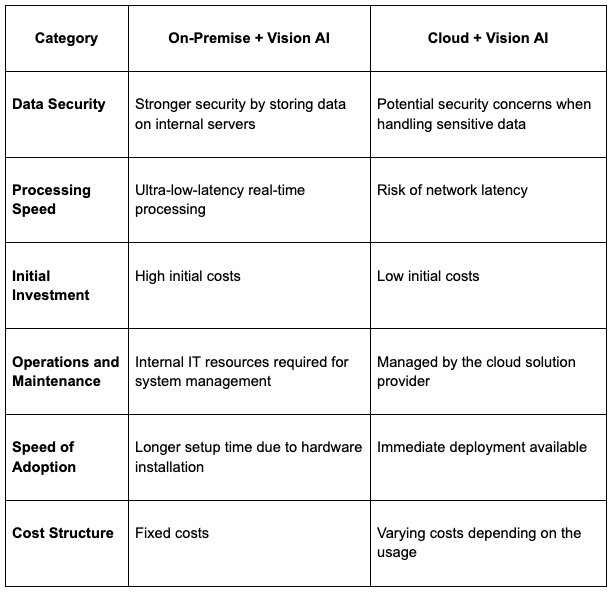
Conclusion: Can We Expect an On-Prem Solution Without Management Burden or a Cloud Solution That Ensures Real-Time Processing Without Security Concerns?
Because Vision AI requires large-scale data processing and high computational performance, selecting the right platform demands a strategic and well-informed approach. To address this need, Superb AI supports digital transformation with a comprehensive approach, offering on-premise, cloud, and hybrid solutions tailored to diverse business requirements.
What if on-premise is the only viable option due to site conditions, but managing continuous operation and maintenance is difficult due to IT resource constraints? – Superb AI experts can provide the solution.
When large volumes of sensitive data requiring strict security measures are continuously generated, it is also possible to train and deploy AI models in real-time while maintaining data security. Superb AI offers flexible solutions tailored to each company's data security, cost, and technical requirements.
In the next post, we will take a closer look at Superb AI’s on-premise and cloud solutions, exploring their key features and benefits.
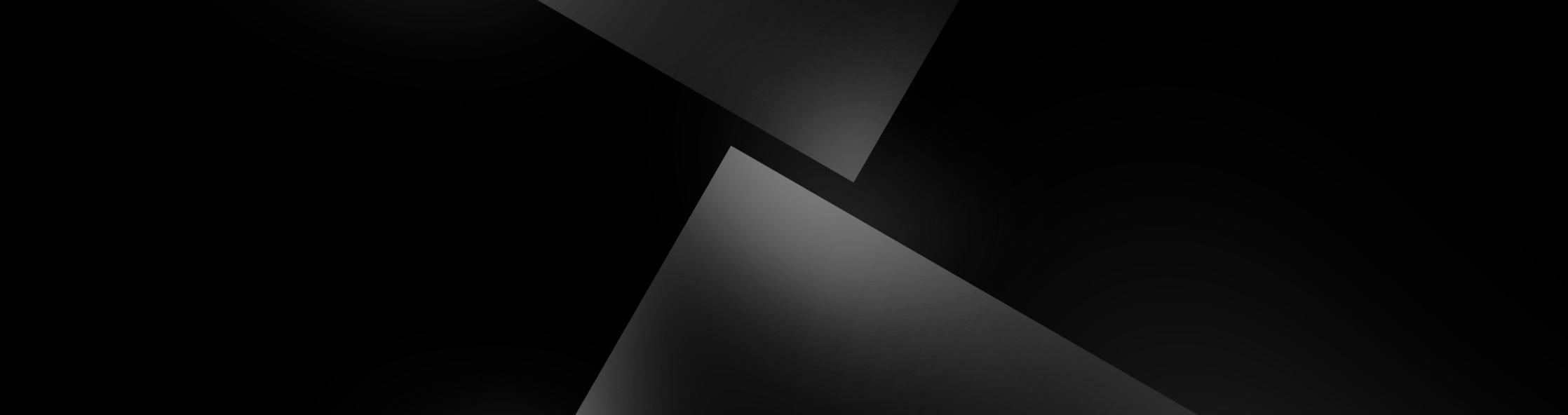
About Superb AI
Superb AI is an enterprise-level training data platform that is reinventing the way ML teams manage and deliver training data within organizations. Launched in 2018, the Superb AI Suite provides a unique blend of automation, collaboration and plug-and-play modularity, helping teams drastically reduce the time it takes to prepare high quality training datasets. If you want to experience the transformation, sign up for free today.